Breaking Free from MRP: Simplifying Manufacturing Workflows
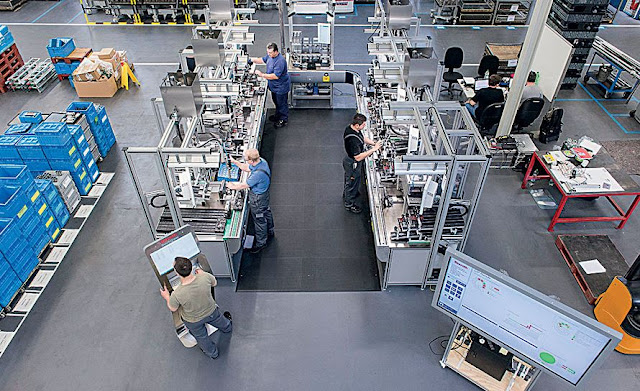
Material Requirements Planning (MRP) is a crucial aspect of supply chain management, as it helps organizations to efficiently manage the flow of materials and ensure that the right amount of raw materials, components, and finished products are available when required. However, MRP comes with its own set of challenges, which include significant configuration needs and administrative overhead. These challenges can be addressed by implementing a smart min/max planning setup and accompanied by a full featured work order management system. One of the major challenges of MRP is its significant configuration needs. MRP requires a considerable amount of setup and configuration, which can be time-consuming and complex. For example, an organization must first set up its bill of materials, routing, and inventory parameters to ensure that the MRP system accurately calculates and forecasts the demand for materials. Fu